Predicting a Student’s Characteristics from a Chatbot Conversation
Agnes Pakozdi • May 13, 2021
Introduction
Universities from all around the world continuously develop Intelligent Tutoring Systems to address the need of individual learning experience and to ease the tutoring burden of teachers and tutors. The Covid-19 pandemic further accelerated the need for digital solutions in education.
Just before the Covid pandemic, in 2019, a group of teachers and researchers from the Business Information Systems (BIS) master program at the University of Applied Sciences Northwestern Switzerland (FHNW) came to a decision that they will develop a chatbot Intelligent Tutoring System (ITS) to support BIS students working on their group assignment. This is an ambitious endeavor and developing such a system requires a lot of resources and preliminary research before the actual chatbot and system development starts. It was important to conduct a study in advance as a first step to understand if such an ITS would really help individual students while working on their group assignment and serve their tutoring needs. This blog post explains the goals and results of this preliminary study that was originally published in the form of a master thesis.
Why do we need to predict the student’s characteristics for an ITS?
During the conventional tutoring process, teachers are able to make assumptions about the characteristics of the students and their knowledge already when they sign up for tutoring. This perceived knowledge about the student’s characteristics continuously changes in the tutor’s mind as the tutoring progresses. Finally, teachers are able to suggest future learning activities based on how they assessed the student at the end of a tutoring class.
An Intelligent Tutoring System (ITS) also needs to build up this knowledge about the student’s learning progress to suggest proper individual tutoring steps for the student.
But wait! How can a system assess students’ characteristics?
An ITS can make predictions about the student’s learning progress from their behaviour with the system. For example, how the student communicates with the system, what words they use, how many failures they make during an assessment question, or if they are proactive in a conversation. These systems model the student’s characteristics in the so-called student models. These student models are a very important part of an ITS, that usually consists of 4 elements; a domain model, a tutoring model, a student model, and an interface. The picture below shows the standard architecture of an ITS with the four components from Nkambou et al (2010).
In this architecture, the domain model contains all knowledge elements of the field to be studied. The student model can be described as a dynamic model that contains information about the student’s intellectual and emotional state during the learning process. The tutoring model makes an assessment based on the information coming from both student and domain models and suggests tutoring actions. The interface component ensures access to the tutoring system (Nkambou et al., 2010). In our research we focused on the student model of a future Intelligent Tutoring System at FHNW.
There are several approaches on how to build student models in Intelligent Tutoring Systems. Stereotype models, machine learning models, cognitive approaches, fuzzy logic or Bayesian Network models can be used to build student models, just to mention a few (Chrysafiadi & Virvou, 2013).
As a student model, we built a Bayesian Network, called DigiBnet, to predict the student’s characteristics, such as their knowledge and motivational level from an imitated chatbot conversation.
Why a chatbot ITS?
The Digitalisation of Business Processes (DigiBP) module from the BIS Master program was selected to be the first module where the study program would offer tutoring via a chatbot Intelligent Tutoring System, called Digital Self Study Assistant (DSSA). The DigiBP module was selected, because it was observed during the years that students need more tutoring to complete their group assignment compared to other modules. Furthermore, the tasks of the assignment are often non-equally divided, as students with programming skills have higher workload compared to non-programmer students. Thus, non-programmer students have difficulties reaching their study goals.
After the module was selected, we conducted a preliminary survey among the DigiBP students to understand if they have a special tutoring need compared to what we already found out from the literature review and if they would positively accept a chatbot tutor. The positive acceptance of chatbot tutors was confirmed by this preliminary survey and we could also find out the special tutoring needs of DigiBP students.
In addition to that survey, we could learn from previous studies and research how student models can be deployed behind conversational agents and chatbots.
Kerly and Bull (2006) were the first researchers who proved the positive acceptance of a possible chatbot interface for a special type of student models, called open learner models, with whom students can negotiate their knowledge via a chatbot interface. After they built the CALMsystem, Kerly and Bull found that the system not only improved the users’ self-assessments accuracy but helped them to stay responsible with their learning process by being challenged by a proactive tool, like a chatbot that they were willing to work and communicate with.
Why Bayesian Network for the student model?
After considering several previous works, we decided to build a Bayesian Network open student model for the future DSSA. The lack of data and information about students, and the high uncertainty drove us towards this approach. It seemed that only this method could ensure that we can make predictions about the student’s characteristics from human experts’ insights and define some prior probabilities in a Bayesian Network (Duda, Hart, & Nilsson, 1981). This approach seemed feasible to determine conditional probabilities intuitively (Conati, 2010) and to calculate the tutoring needs of the students.
Bayesian Networks need input variables from different observations to predict a certain event. To find the right input variables for our student model Bayesian Network, DigiBnet, we used some findings from Hill’s research (2015) that reveals differences between human-human and human-chatbot conversations. As an addition to Hill’s research, an anonymized chatbot answer data was used from the Business Intelligence (BI) module from the Autumn Semester 2019 at FHNW for further inspiration to define possible observation inputs.
Based on these above mentioned insights we have considered the length of messages as an indicator of student motivation (where very short messages usually indicate low motivation), the number of knowledge elements (correctly) covered in the conversation and the number of questions and hints that the chatbot had to ask to achieve that coverage. In the picture below, the mentioned aspects can be seen as orange nodes in the Bayesian Network. It also shows how DigiBnet predicted the tutoring need of a student based on the activated input variable (orange) nodes.
From the activated input variables the Bayesian Network could predict the knowledge and the motivational level of the students, from which the final tutoring need of the students was further predicted by the model.
How did we conduct and evaluate the chatbot conversations?
To test the DigiBnet student model, we have imitated 4 chatbot conversations with students with assumed different knowledge levels in the topic. One teacher took over the role of a chatbot who followed the dialogue policy (not described in depth here) and responded to students’ utterances as if the answers were given by a chatbot. Students were informed that they were interacting with a teacher who took over the role of a chatbot and were also asked to give answers as if they were communicating with a chatbot. Thus, the research was not a blind Wizard of Oz study (Dahlbäck, Jönsson, & Ahrenberg, 1993), but an instant messaging chat between student and teacher. Although this setup might have caused some biases in our research, this method seemed the most feasible to test our Bayesian Network student model with the available resources to conclude some initial learnings.
After we conducted the chatbot conversations with the students, we used the chat text files and manually evaluated the observations that were needed for DigiBnet to activate its nodes. Based on this input data, our student model could assess the knowledge and motivational level of the students and could calculate the probabilities of which tutoring advice would serve the student’s need the best.
After the conversations were evaluated, we conducted interviews with the 4 students and shared with them how DigiBnet assessed their knowledge and motivational level, and what kind of tutoring it would suggest to them.
What did we find out from the interviews?
When we shared DigiBNet’s assessment with the individual students, there was only one contradiction where one student did not agree with DigiBNet’s assessment. All other assessments of the DigiBnet were correct and accepted by the students. It means that the observations that the model used for prediction and the assumed conditional probabilities were capable to properly predict the knowledge and motivational level characteristics of the student.
From earlier research we know that opening the student model and sharing the model’s assessment with the students can improve the metacognitive skills of the students, which results in better student performance (Mitrovic & Martin, 2002). We could also confirm that, as a result of the student's self-reflection that they would be grateful for advice regarding the need to study further materials. Some of the students found that they received rather general than personalised tutoring advice, i.e. it did not go much beyond what they already knew. We could conclude from the feedback that the well formulated and placed advice is useful for the students, but it should be provided during the tutoring conversation, after it is clear for the model that the student doesn’t know the answer of a specific question. Students suggested additional video tutorials showing the exact minute of the video, where the missing part is explained in detail, or a particular paper or blog post directing the students to the questioned topic of the material would be highly useful for them.
What are the key learnings?
From our research we concluded that there is a need from the students for personalised tutoring advice. From our preliminary survey we found that most of the students (79.5%) are open to communicating with a chatbot about their assignment and would positively perceive an assessment from a chatbot (72.7%). DigiBnet could predict the characteristics of the students well and they were positively accepted by the students. Providing individual tutoring however requires more fine tuning and testing to find out what would be really beneficial for the learners.
Building a chatbot ITS is a very complex journey with several modelling, testing, prototyping tasks before we start building a knowledge base and developing a system. In our research we proved that such a system would be beneficial for the students, however it is still a long journey to put these systems into production at FHNW. The first steps are done, let’s see what comes next.
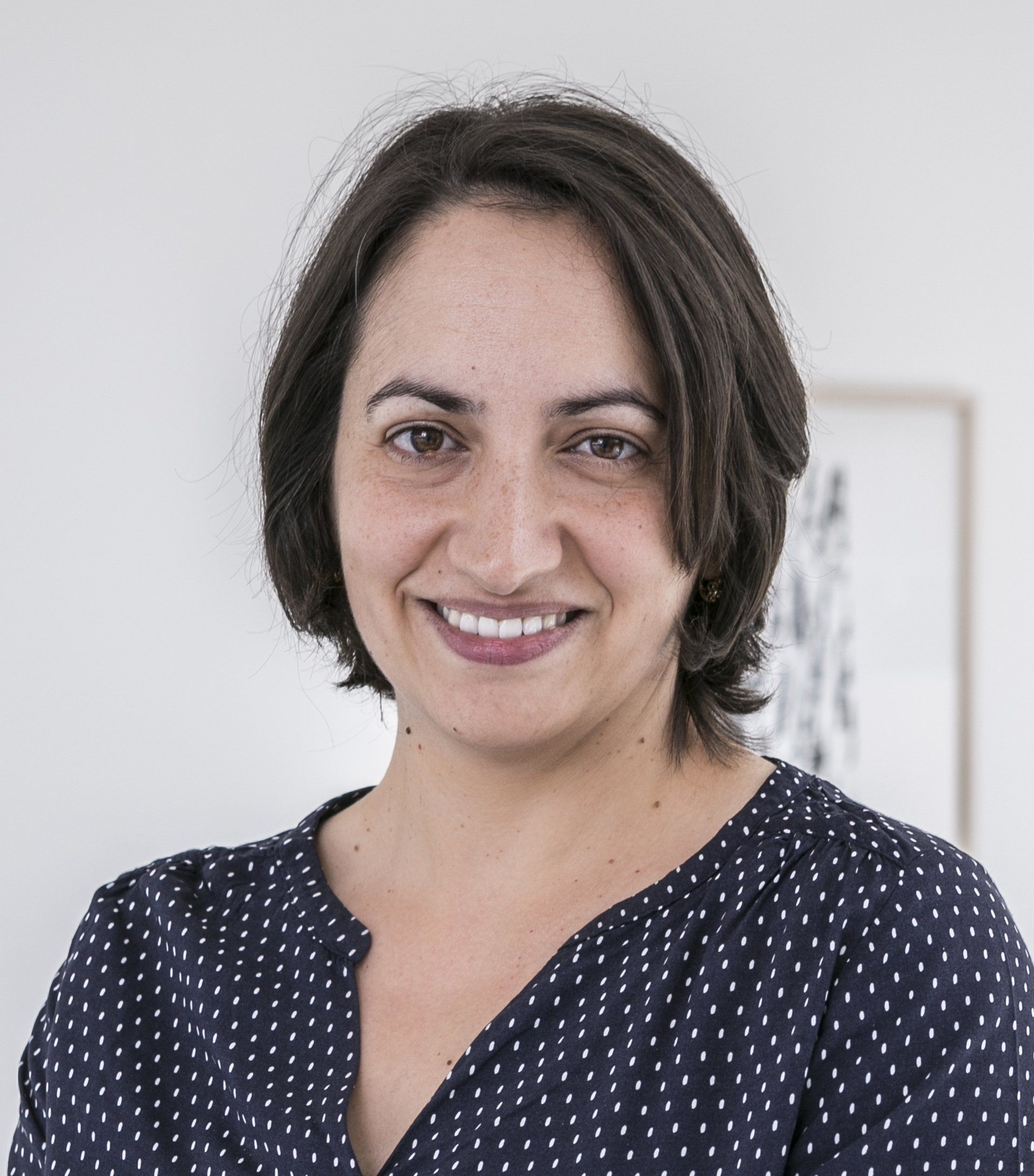
Agnes Pakozdi
Business Analyst, Zühlke Group
Agnes Pakozdi has finished her Master of Science studies in Business Information Systems at FHNW under the supervision of Dr. Hans Friedrich Witschel in 2020. Agnes also holds a Bachelor diplom from Budapest Business School, a Certificate in General Management from HSG and a Diploma in Product Management from Product Academy. Agnes is passionate about digitalisation, product management and connecting Data/IT with business.
Need support with your Generative AI Strategy and Implementation?
🚀 AI Strategy, business and tech support
🚀 ChatGPT, Generative AI & Conversational AI (Chatbot)
🚀 Support with AI product development
🚀 AI Tools and Automation
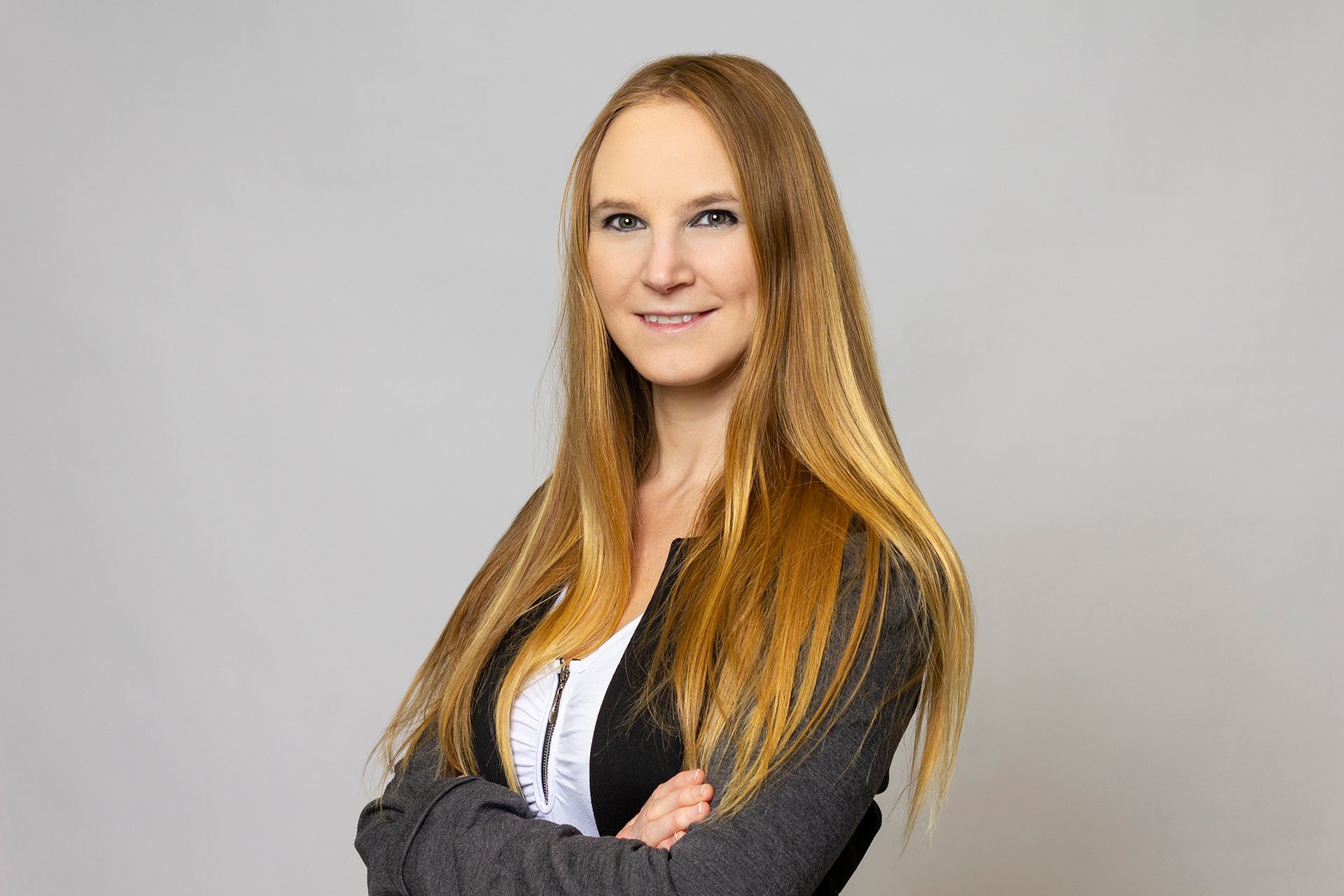
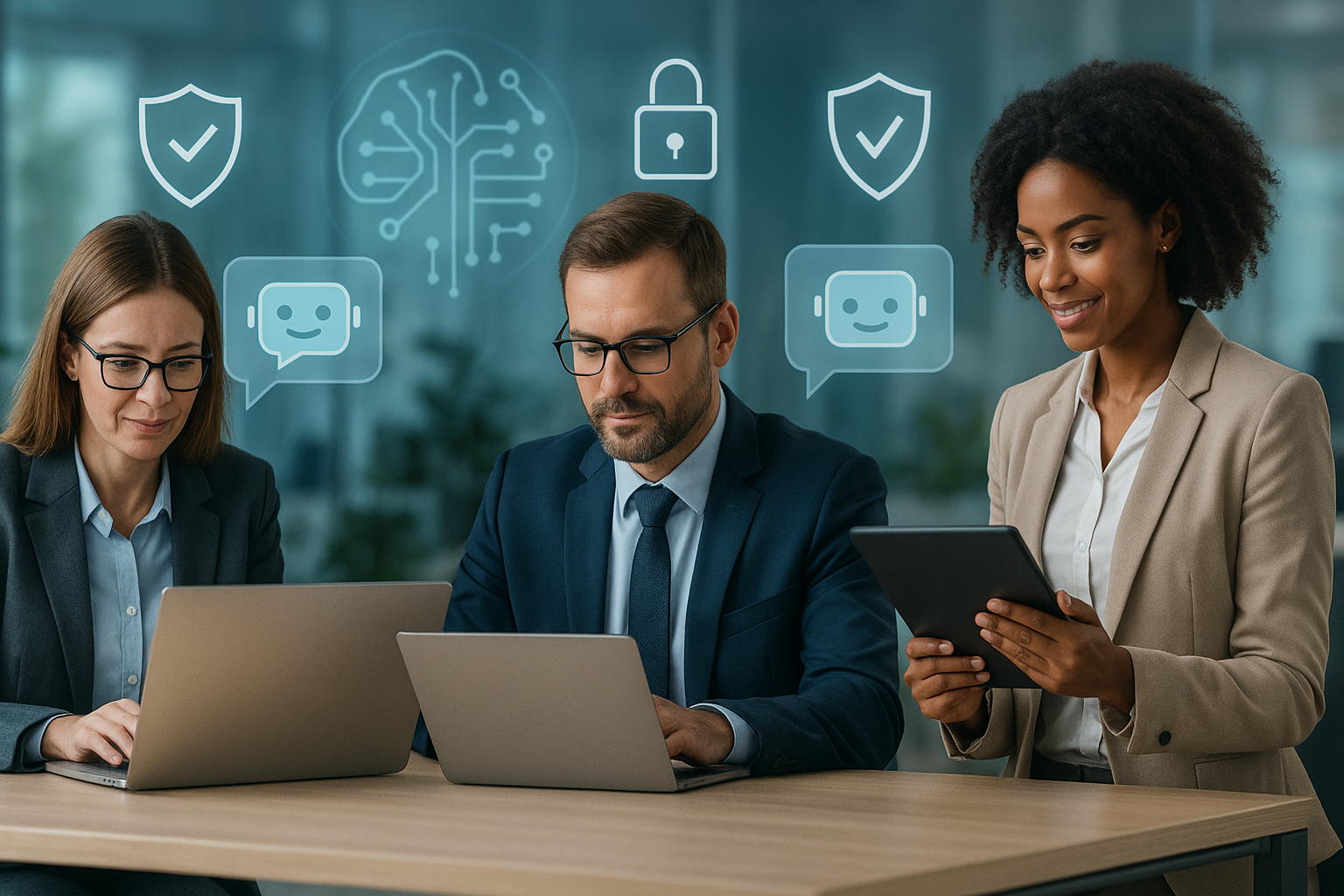
AI-powered chatbots, whether developed in-house or deployed through trusted platforms, are revolutionizing customer service, knowledge access, and internal communication. However, alongside these opportunities come new legal obligations: data protection , transparency , and EU AI Act compliance must be addressed carefully. This article covers: Where AI chatbots bring business value What compliance risks you must manage How to implement AI chatbots successfully and securely
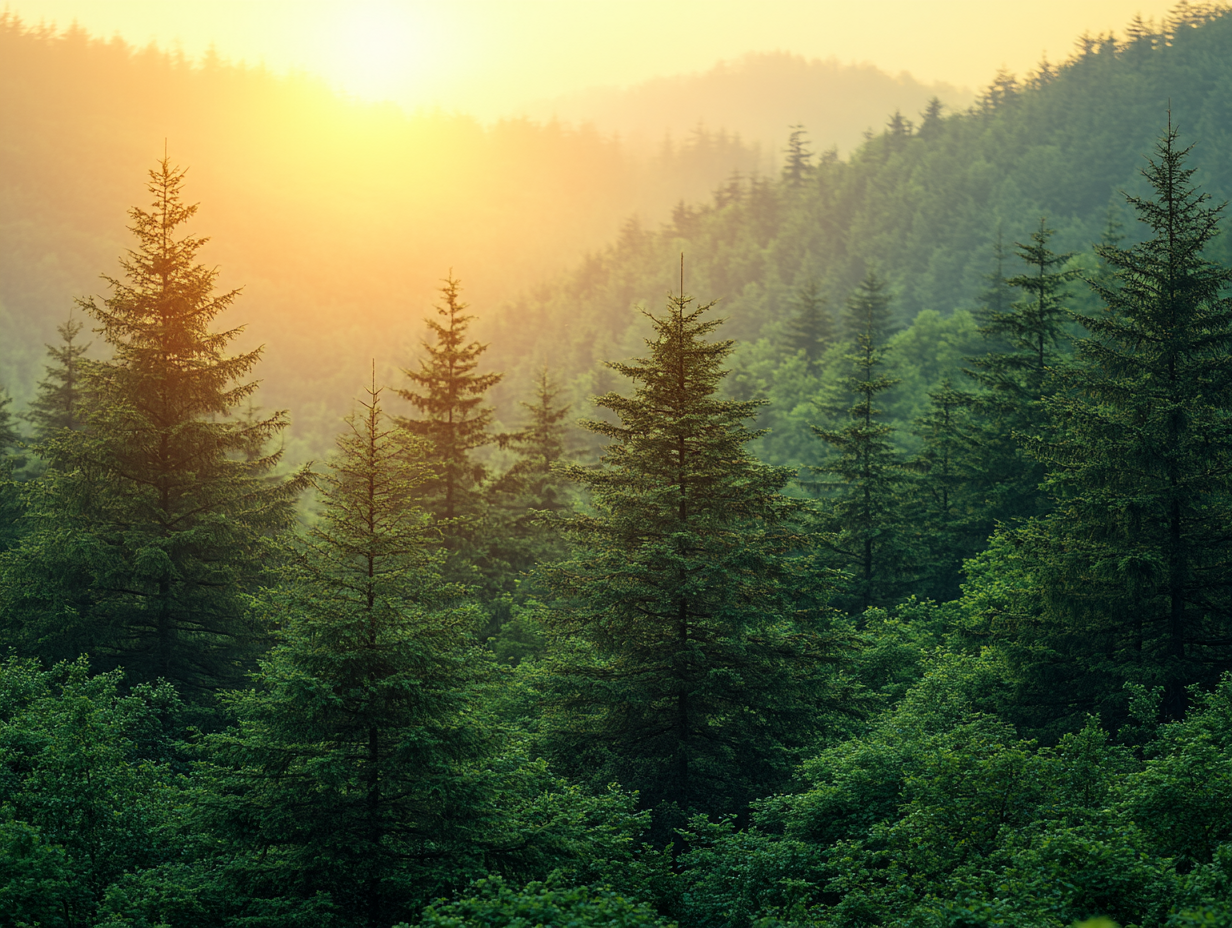
Since August 2024, users have been able to use the web version of the image creation tool Midjourney. This simplifies usage by providing a user-friendly interface to experiment with one of the top Generative AI image creation tools available. We tested it for you and are sharing helpful tips and tricks. How to prompt images with Midjourney? If you use Midjourney on discord, there is a clear prompt structure and prompt parameters to adhere to. Usually, it makes sense to stick to it: 1) To prompt use "/Imagine" 2) Then enter your subject (description and details) you want to see on the image and it's environment (see yellow highlighted below in the prompt example) 3) Then enter composition, lightning, colours (see green highlighted below in the prompt example) 4) Finally add technical parameters to adjust and finalize your image. Please find a useful parameter library here.