How Generative AI can Boost your Company Page Search
With the advances in Generative AI companies should consider how to be "on top" when it comes to new technologies such as ChatGPT and Generative AI assistants. While Google SEO was one of the main drivers before the launch of ChatGPT by OpenAI, today companies should optimize towards the next-generation of search engines based on large language models and advanced search models.
Why are Generative AI searches so intelligent?
Intelligent searches basically understand the semantics in a sentence. So old index-based searches used to be sufficient for keyword entries but did often fall short when users did not enter exact the correct keywords or did spelling errors. Also AI based searches with older NLP classification models before the launch of OpenAI were handling some of these challenges but did never achieve the level of intelligence of so-called embeddings. With new vectorbased embeddings (which are also available by OpenAI) companies can build intelligent searches that can give to their users the best solution across all their websites.
What are embeddings?
Basically you can think of a vector representations. A user question is translated into a vector representation and this vector is used to query a vector database. Then the answer is used do create a summary via llms.
Think about when your kid plays with its toys. It might group them together based on what they are or how to use them. Your kid might put all your cars together because they all have wheels and can move around. Then it might put all its action figures together because it can play make-believe with them. And the kid's board games would be in another group because it plays them on a table. This is similar to how vector embeddings work in computers. But instead of toys, we have words. Just like how your kid groups toys, a computer groups words that are similar. For example, words like "cat", "dog", and "hamster" could be in one group because they are all pets. But how does a computer know which words are similar? It learns from reading a lot, like how you learn from playing and studying. If the computer sees the word "dog" being used in similar places as the word "cat", it will think these words are related and put them close together in its group.
So, in the end, vector embeddings are like a big, organized toy box for a computer, but with words instead of toys. Just like how a kid can more easily pick a toy to play with when its toy box is organized, a computer can more easily understand and use words when they are nicely grouped by vector embeddings.
How can companies create their own Generative AI search?
OpenAI did not only change the way companies could search with ChatGPT but it also changed the way companies can create their own page search. There are several paid and open-source models (embeddings from HuggingFace or OpenAI and Meta) to create your own intelligent search. If you need support with the development of your own search contact our team for any support.
This graph from TheAiEdge.io nicely illustrates how embeddings work:
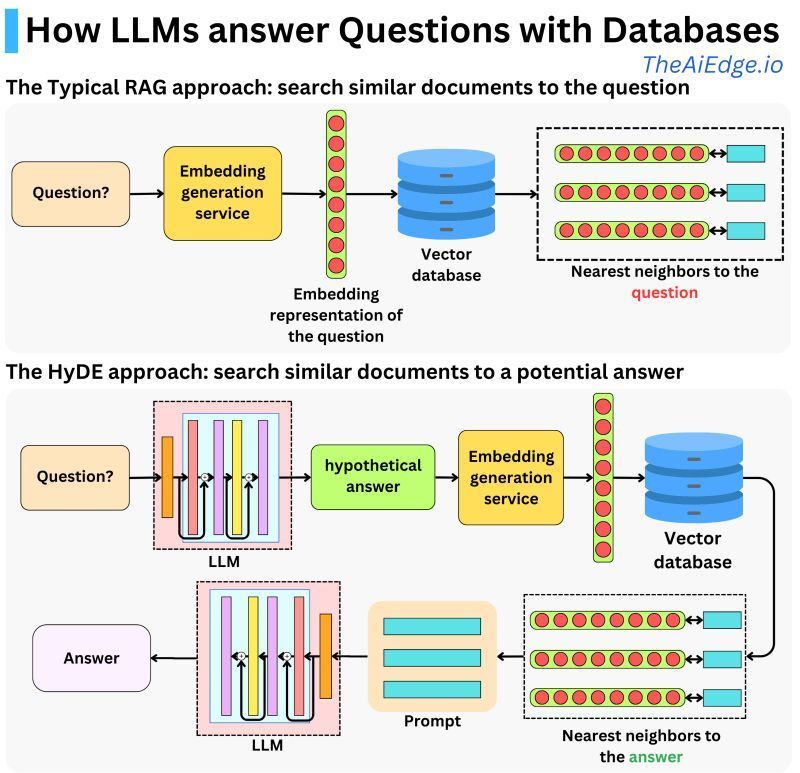
Need support with your Generative AI Strategy and Implementation?
🚀 AI Strategy, business and tech support
🚀 ChatGPT, Generative AI & Conversational AI (Chatbot)
🚀 Support with AI product development
🚀 AI Tools and Automation
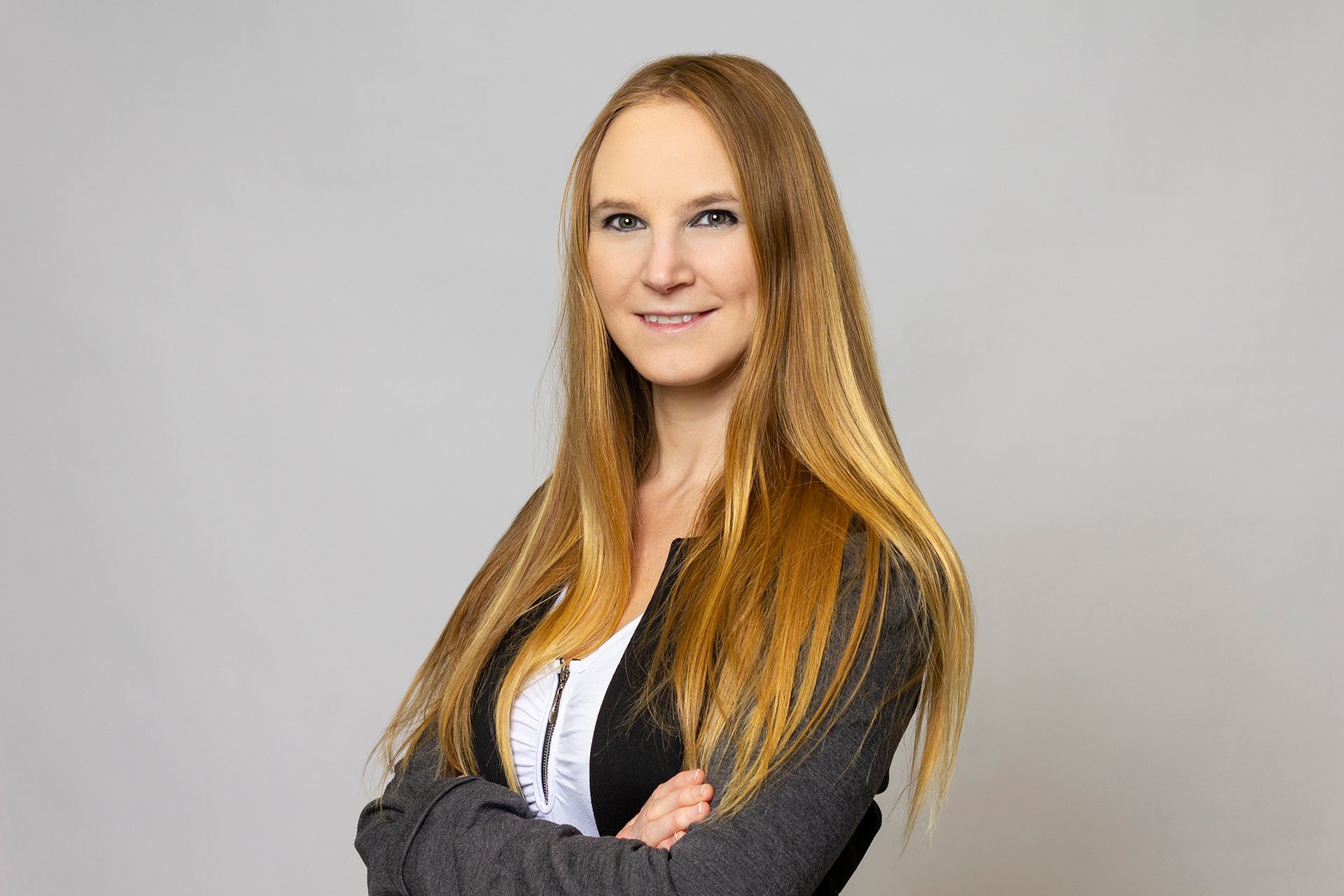
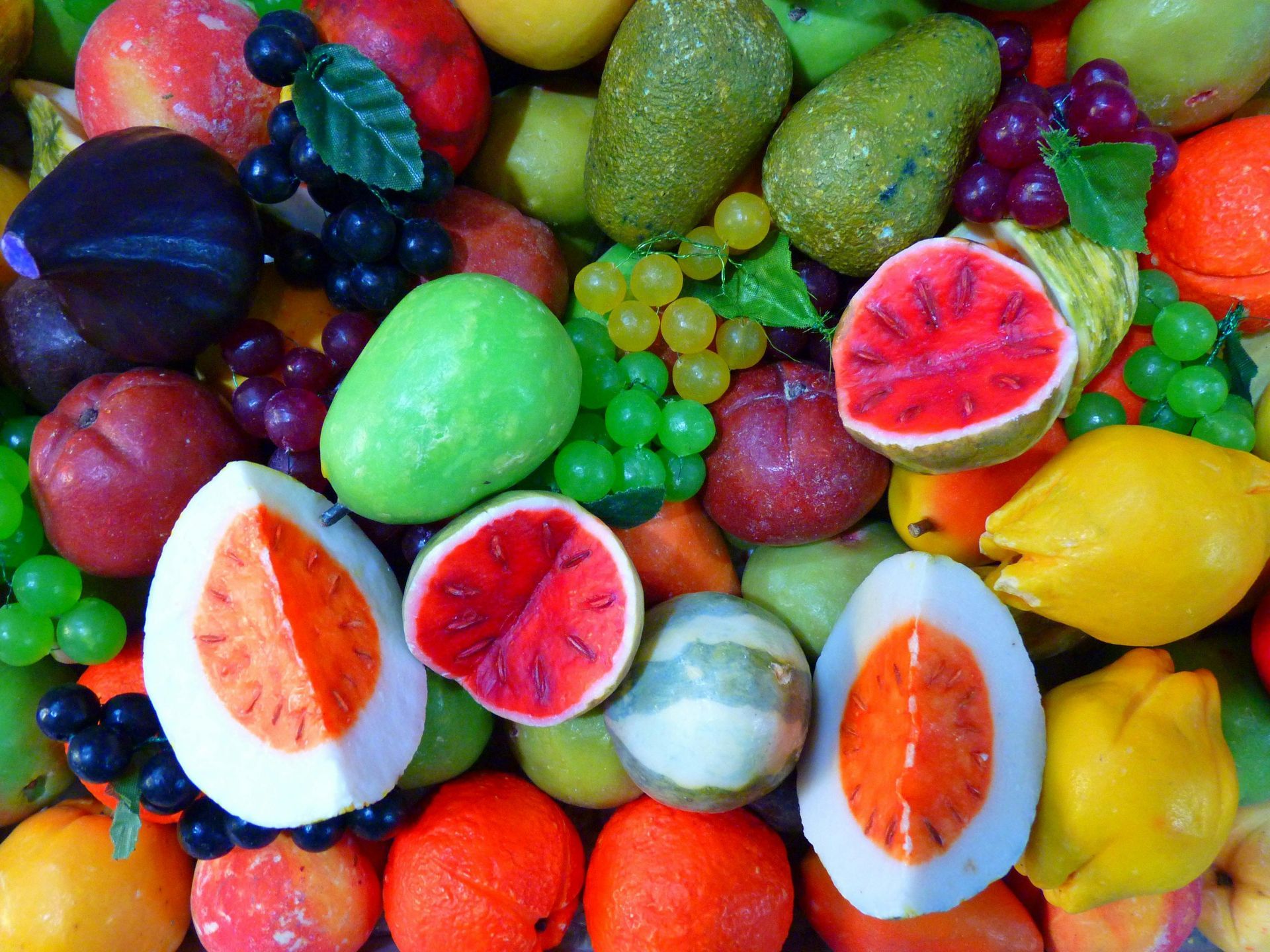
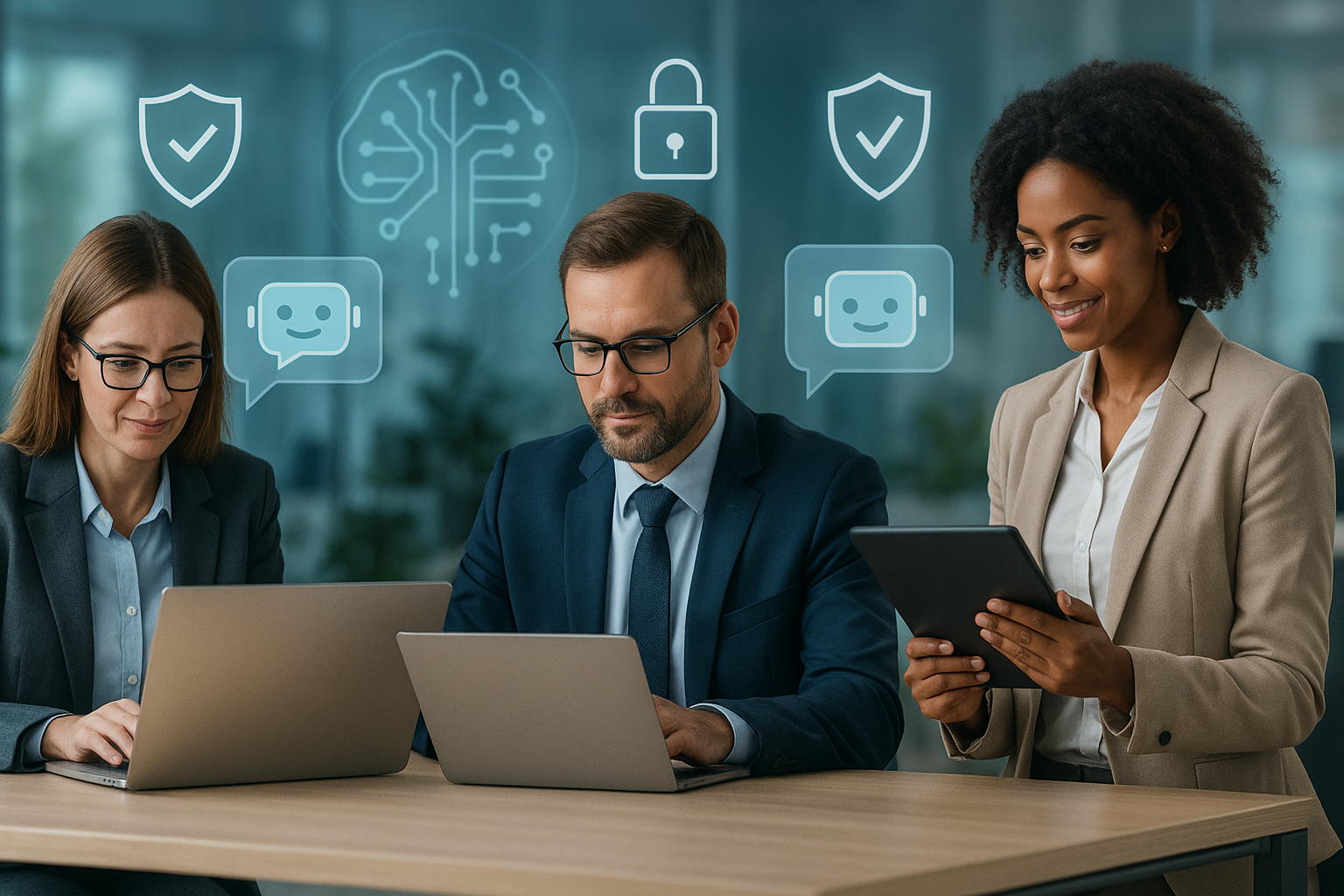
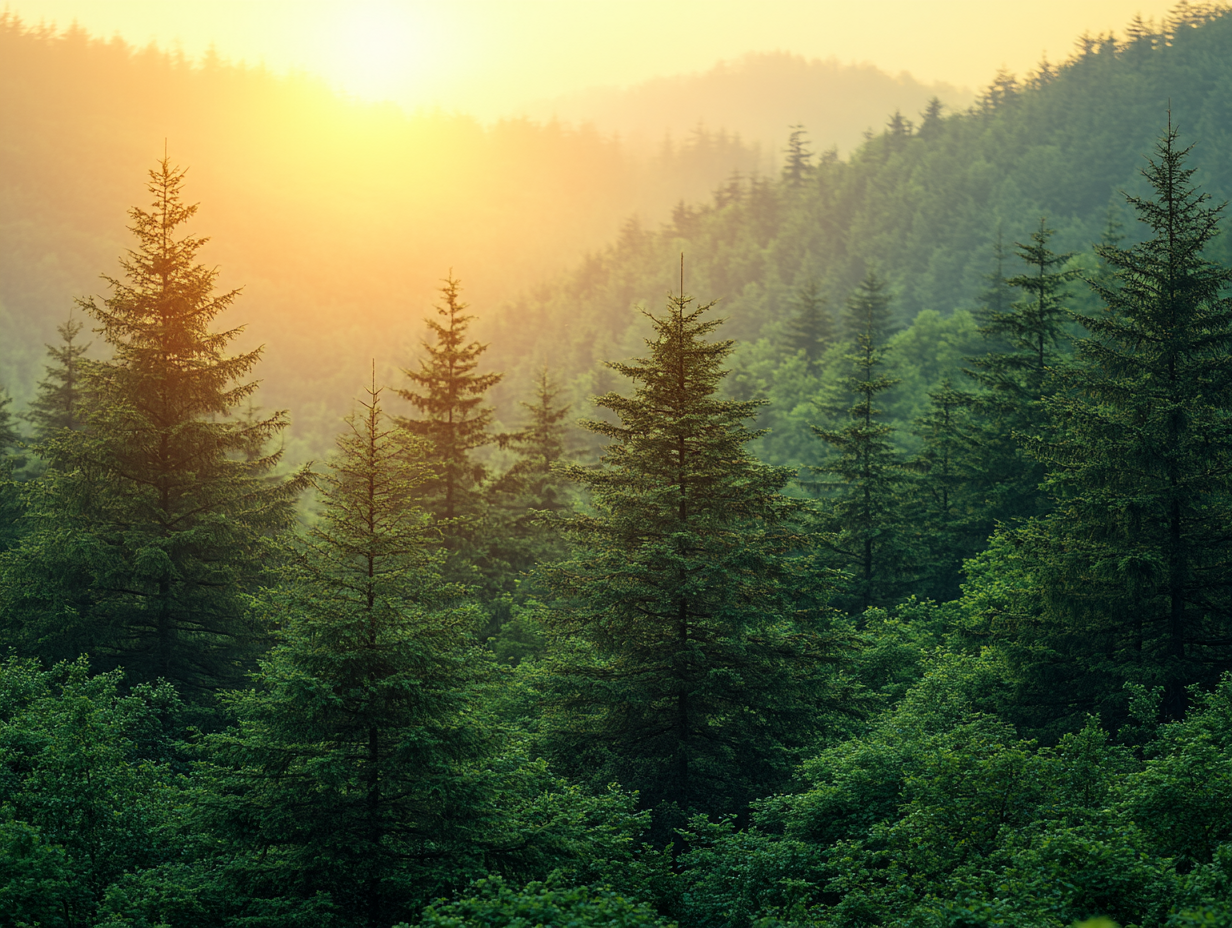