What are the costs for enterprises to use llms?
Many companies are reluctant when implementing llm-based products because they fear bein confronted with high costs. Especially for medium-sized companies which have not the ressouces or enough capacity to deploy and oprimize their AI models nor to set up an own infrastructure with MLOps. As described in our article about sustainability of Gen. AI applications, cloud and performance costs of running an llm can become very high.
What are the cost types when implementing OpenAI or other llms?
There are four types of costs related to llms:
- Inference Costs
- Setup and Maintenance Costs
- Costs depending on the Use Case
- Other Costs related to Generative AI products
What are inference costs?
An llm has been trained on a huge library of books, articles, and websites. Now, when you ask it something, it uses all that knowledge to make its best guess or create something new that fits what you asked for. That process of coming up with answers or creating new text based on what it has learned is called inference in LLMs.
Usually, developers would call a large language model like GPT-4. But here comes the "but": usually not only large language models account to the total costs when running the final product. To explain: LLMs can be used to classify data (e.g undestand that the text talks about "searching a new car insurance"), for summarization, for translation and for many other tasks. Download the ultimative Gen. AI Task Overview to learn where llms make sense:
What are the cost factors affecting the LLM costs?
Factors affecting LLM cost include model size (e.g. how many parameters it uses), with larger models requiring more resources, and context length (how much data, respectively text you provide to the model when you request your question), impacting computational demands. Keep in mind that larger models are not always better.
- Enterprises face a range of choices between proprietary models like OpenAI's and open-source models like Llama 2 and Falcon 180-B. The selection of an LLM ifself depends on specific use cases and a balance between cost and performance.
Which Generative AI use cases cost more?
As outlined in our "Tasks where Generative AI helps" framework we show different use cases where generative AI makes sense.
- Cost vary especially with the use case. For tasks like "summarizing" GPT-4 is often preferred in researches but comes with high costs at OpenAI.
- Usually chatbots are more complex and cost-intensive than other Gen. AI products, especially when they do offer more than a "one-shot" response and can "talk for a while" about a topic and hold memory.
- If your information has to be up-to-date at any time to deliver sufficient user-experience and avoid image issues (e.g. healthcare, insurance, fintech sectors), Retrieval Augmented Generation (RAG, see below for more information) costs will be coming on top. These RAG caused daily query costs vary between OpenAI and other models, reflecting different pricing structures.
- Finally, "fine-tuning" is the most expensive process which means you change or customize at least one parameter in your llm neural network. This may change how the model learns on knowledge or its reasoning capabilities. "This leads to $100,000 for smaller models between one to five billion parameters, and millions of dollars for larger models" as outlined in AI Business article. Thus, finetuning comes only into play for large Enterprises or startup that finetune their own large language model or domain (e.g. BloomGPT). In this case, open-source models may lead to less costs in the long-term than OpenAI model finetuning. Keep in mind that people sometimes also refer to "finetuning" related to the optimization of a model concerning its purpose or domain and do not mean refer to any "change the model parameters". The latter is not as expensive as "real finetuning".
How much does a OpenAI model cost per month?
The monthly costs of the model really depend on the model you use, e.g. whether you use GPT-3.5 with 4K context (see below in the table), GPT3.4 with 16K context, GPT-4 with 8K context or GPT-4 with 32K context or even the new "Turbo versions", and on the usage (traffic) of your Generative AI product.
The yearly costs range from $1K-50K on the low usage end, depending on which model. Or from $1M-56M a year for high usage as outlined in the calculation below. Studies show that for low usage OpenAI model make sense whereas for high usage and traffic cases with many millions of users, open-source models can safe costs.
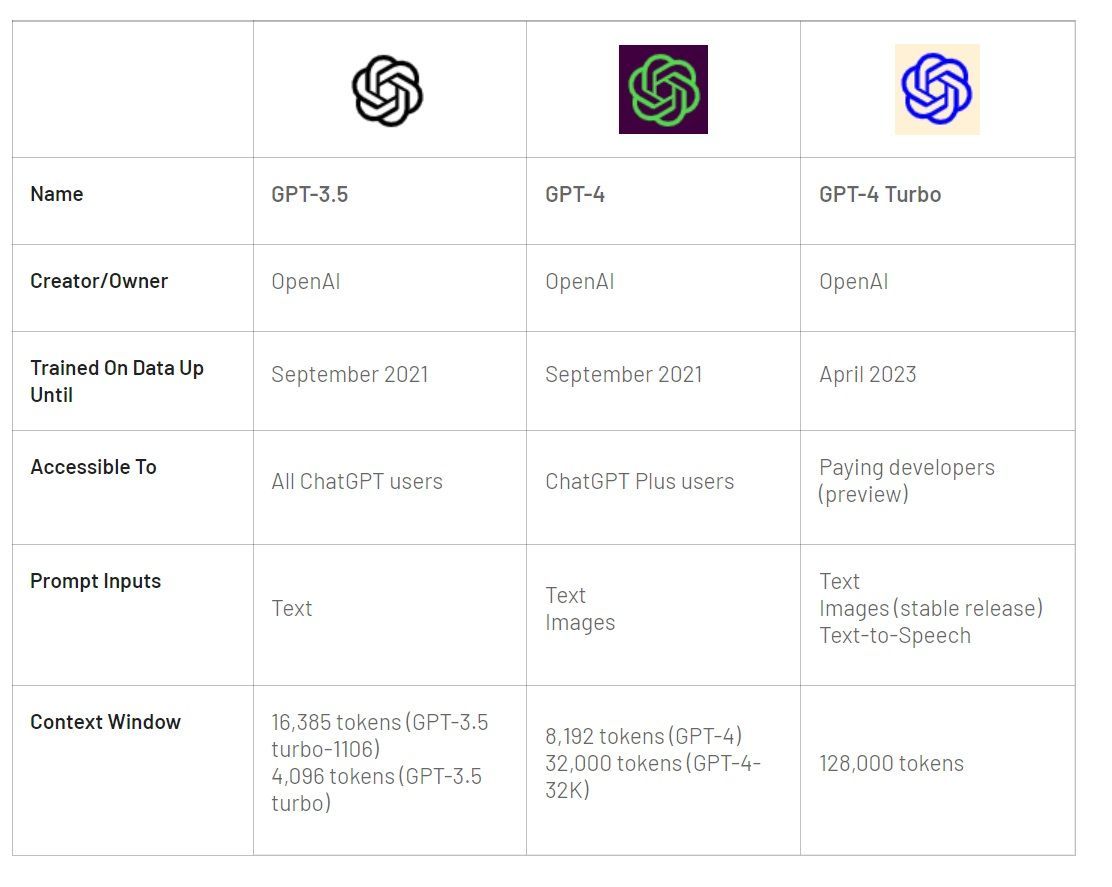
Source: Differences between OpenAI' models. GPT4 is as OpenAI describes "10 times more advanced than its predecessor, GPT-3.5. This enhancement enables the model to better understand the context and distinguish nuances, resulting in more accurate and coherent responses."
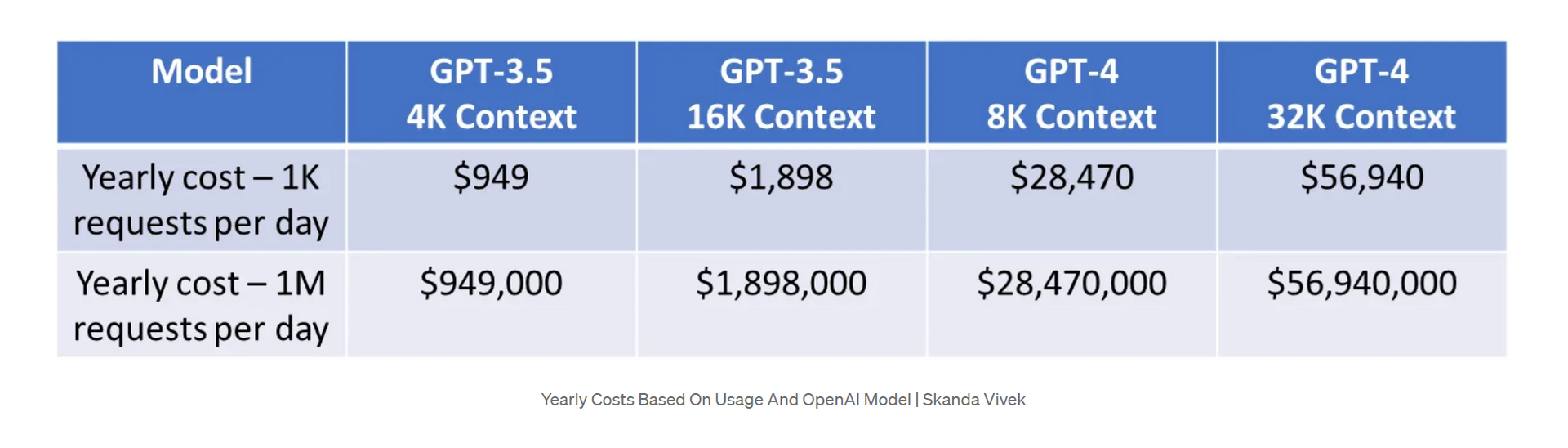
Source: Costs are depending on the GPT model and context as well usage requests. For high traffic applications the authors recommend to use own open-source models instead GPT, respectively OpenAI. Find more calculations here.
What are the maintenance costs related to llms?
To set up the infrastructure for llms you need to consider several points:
First, you need to set up open-source models or proprietary models like GPT on a cloud environment. It is also possible to set up the infrastructure on-premises (where you run your models on private servers) but usually this requires costly GPUs and hardware from providers like NVIDIA. As Skanda Vivek outlines this requires a A10 or A100 NVIDIA GPU. A10 (with 24GB GPU memory) costs $3k, whereas the A100 (40 GB memory) costs $10–20k with the current market shortage in place.
- Compute: FLOPs (floating point operations per second) needed to complete a task. This depends on the number of parameters and data size. Larger models are not always better in performance.
- Data Center Location: Depending on the local data center, energy efficiency and CO2 emission may differ.
- Processors: Computing processors (CPUs, GPUs, TPUs) vary in energy efficiencies for specific tasks
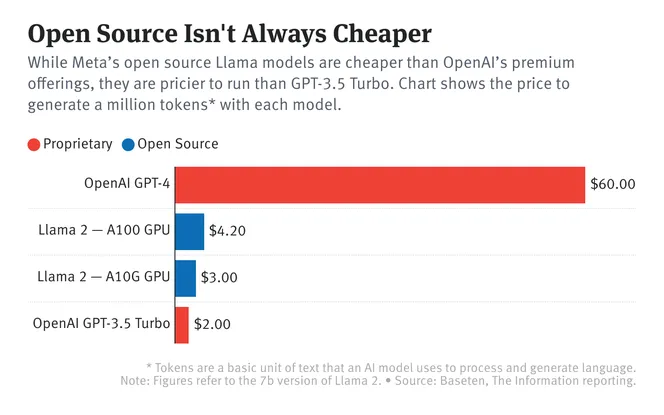
Source: Open Source can become more expensive than GPT-3.5, especially due to complexity in maintenance, prompt engineering and extensive data science knowledge required for non-proprietary models like Llama 2.
What are other costs related to LLMs?
Very often embeddings (vectorization of your text corpus) and RAG (Retrieval Augmentation Generation) are needed besides mere GPT or llm models.
Why? Traditional language models can sometimes make errors or give generic answers because they're only using the information they were trained on. With RAG, the model can access up-to-date and specific information, leading to better, more informed answers. Example: Let's say you ask, "What's the latest research on climate change?" A RAG model first finds recent scientific articles or papers about climate change. Then, it uses that information to generate a summary or answer that reflects the latest findings, rather than just giving a general answer about climate change. This makes it much more useful for questions where current, detailed information is important. For Enterprises, up-to-date content is key, thus there must be some mechanism to retrieve best content continuously.
What is better? Open-Source LLM vs. Proprietary Models, e.g. OpenAI?
This is difficult to say. It depends on your requirements. Please find more in this useful article.
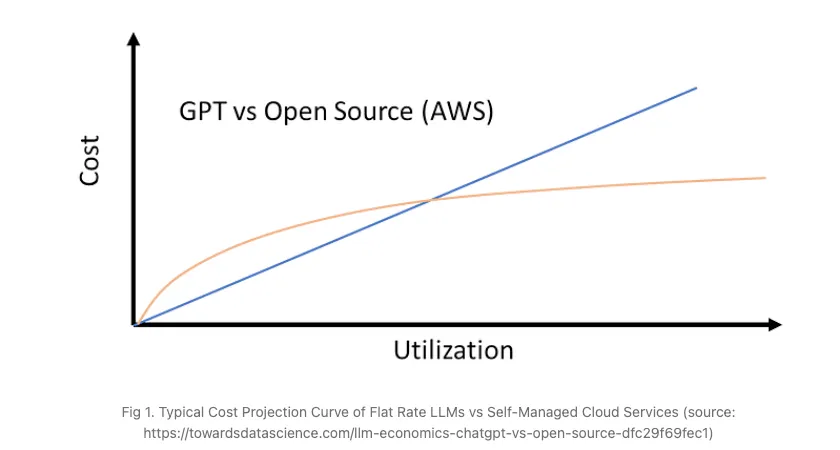
"ChatGPT is more cost-effective than utilizing open-source LLMs deployed to AWS when the number of requests is not high and remains below the break-even point." as cited from the Medium article.
Key Learnings when it comes to the costs for Generative AI products
The costs related llms vary from your use case (chatbot, analysis, voicebot, FAQ bot, summarization, etc.), traffic exposure, performance and set up (on-premises, cloud) requirements.
- Open-Source is often more flexible than OpenAI when implementing own models, with a lot of traffic (> millions of users). However, it requires more prompt engineering, infrastructure customization and data science knowhow.
- Fine-tuning costs differ, with OpenAI models typically more expensive than alternatives like LLaMa-v2-7b.
- OpenAI's models are noted for their higher costs, but being more straight-forward, and efforts like the GPT-3.5 Turbo API aim to reduce prices.
Do you need support with choosing the right big tech provider, Generative AI product vendor or just want to kick off your project?
We are here to support you: contact us today.
Need support with your Generative AI Strategy and Implementation?
🚀 AI Strategy, business and tech support
🚀 ChatGPT, Generative AI & Conversational AI (Chatbot)
🚀 Support with AI product development
🚀 AI Tools and Automation
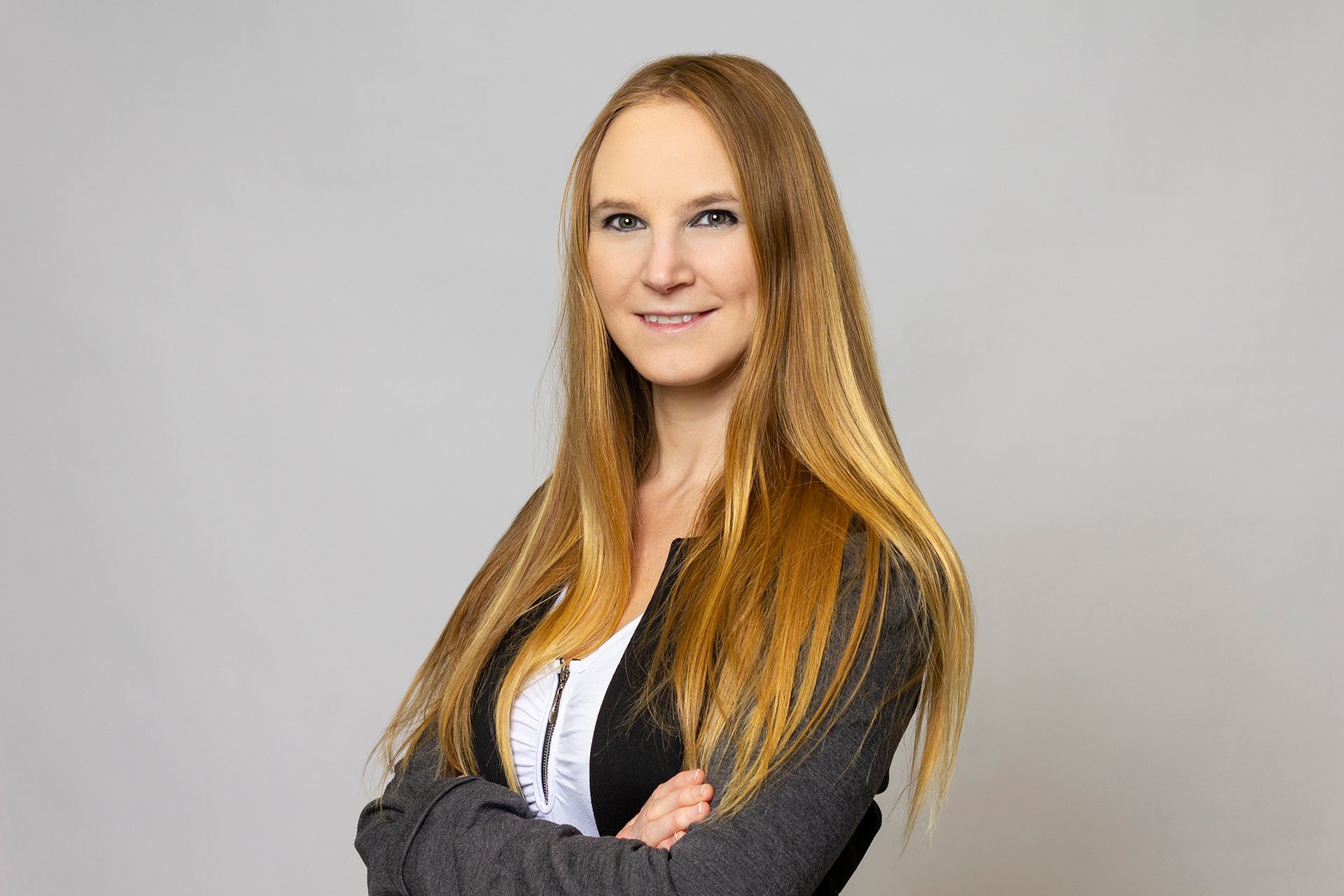
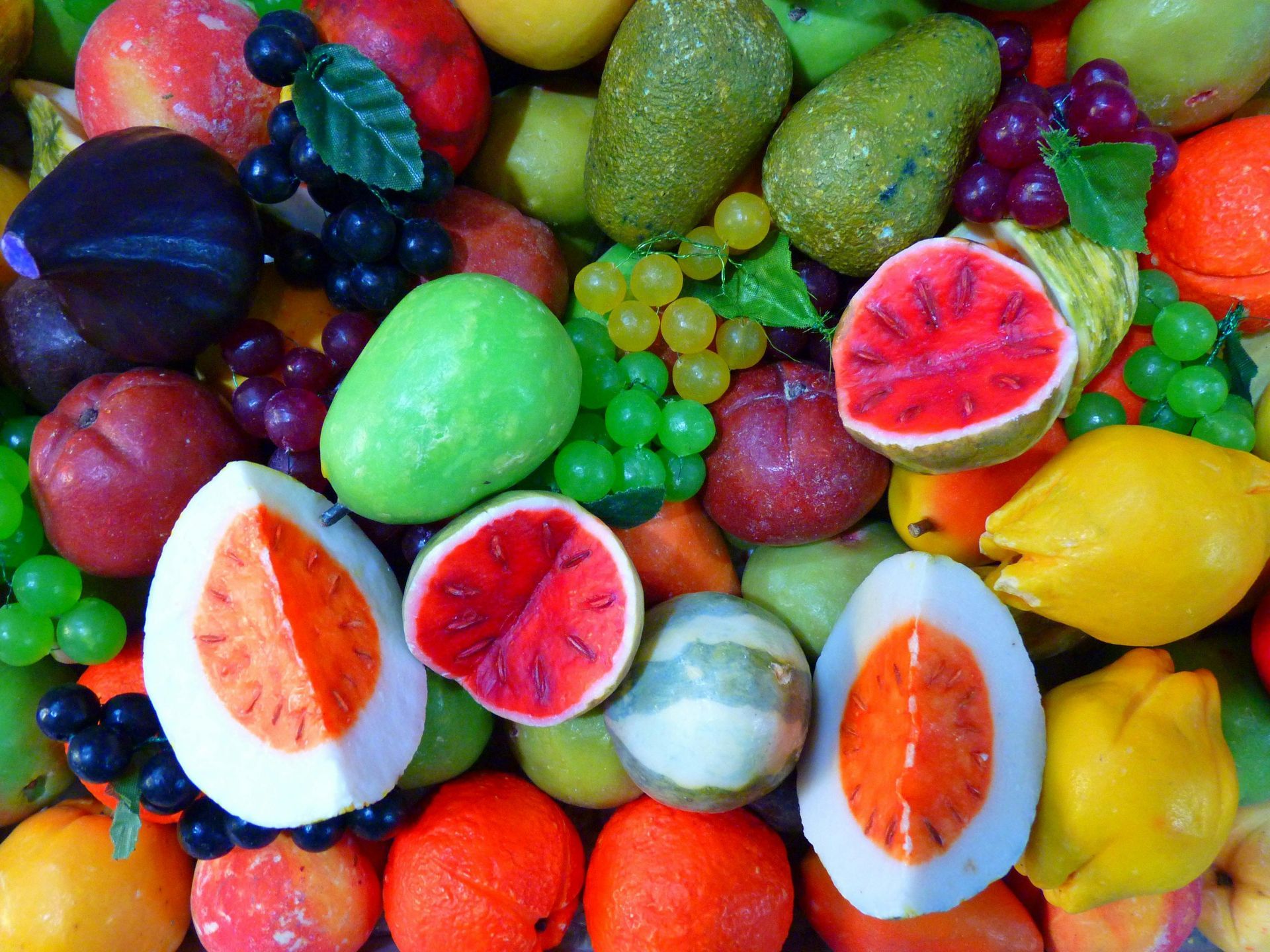
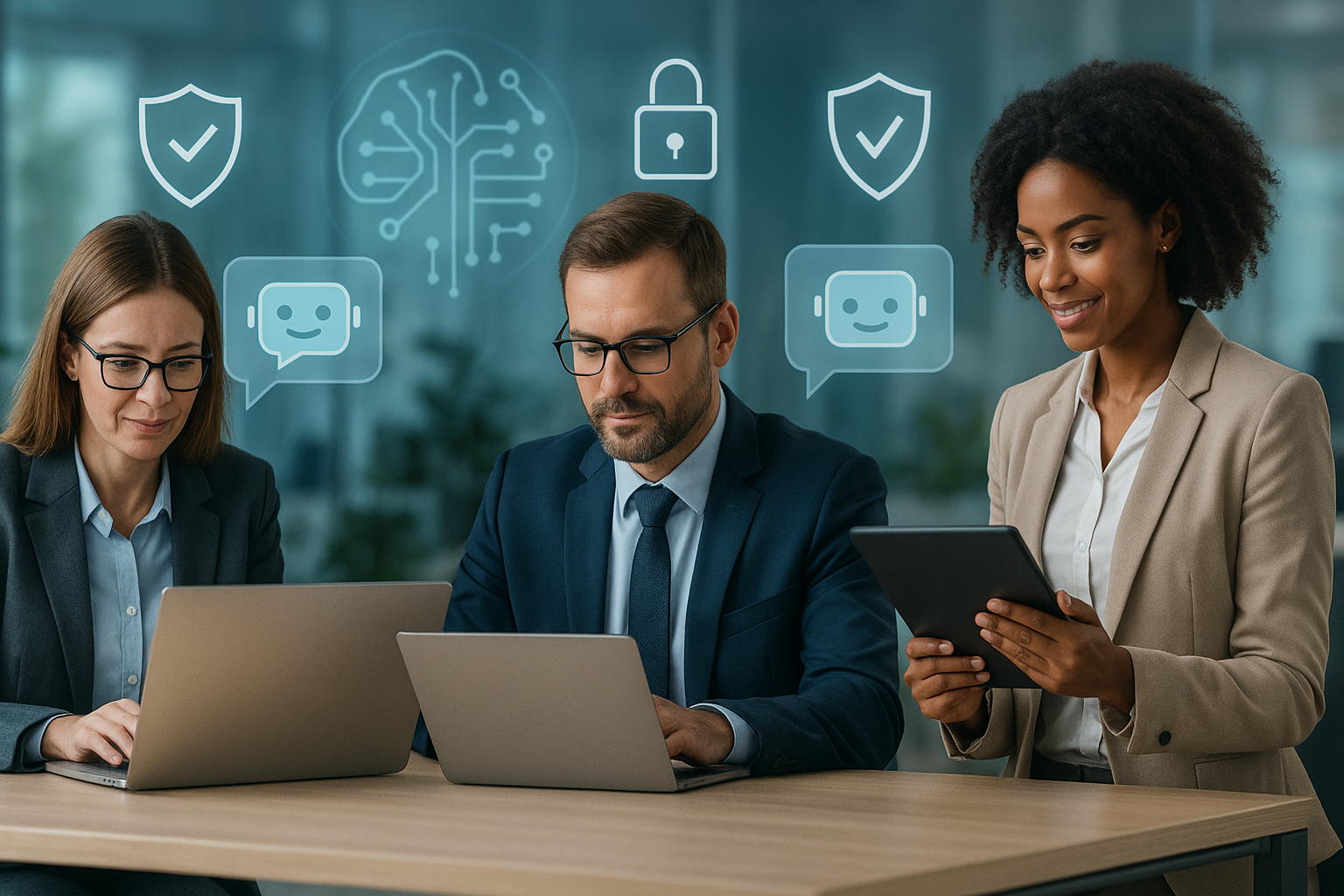
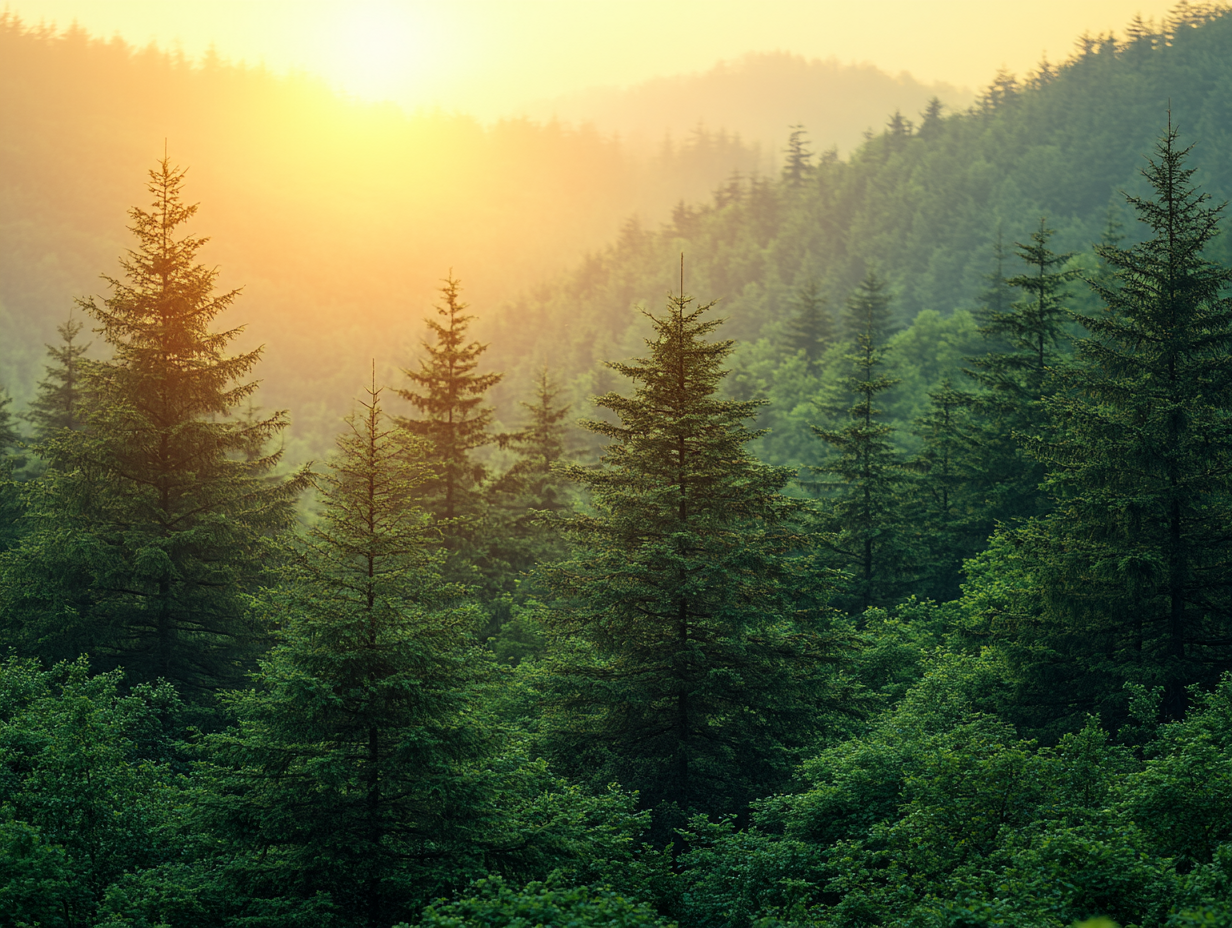