Checklist for companies to successfully implement Generative AI
Often companies struggle to start with Generative AI as there are many unknowns when it comes to the costs, responsible AI and security concerns. Using ChatGPT Plus accounts is fun but of course companies need to be able to establish their own custom environment in order to upskill people and prevent data being sent to OpenAI. As stated in our recent article about ChatGPT and data policy - a simple guide , you need take care when sending questions to ChatGPT as the data may be used for the training of OpenAI's models or provided to third-party vendors.
How to find Generative AI Business Cases?
First, it makes sense to identify the use cases that really make sense to be automized with Generative AI.
Here the Generative AI Checklist that can help you to scale faster. The checklist is built upon of hands-on AI product implementation experience (chatbots, voicebots, NLP search and more) and - unlike other sources - considers all relevant aspects which you will encounter during your AI journey. Take these steps:
1. Customer: Identify the target users and customers you serve with your Gen. AI solution (e.g. internal employees, external clients, new or specific segments?)
2. Pain Point: Write down the top problems or pain points that your target customer faces.
3. Gen. AI Suitability: Check how your product can address the identified pain point and whether Gen. AI makes sense for that.
4. Data and Content Quality: First, you need good data to create a generative AI application.
- Think which data sources are needed for your AI product?
- Where are these data sources in your company? Are there databases, excels, intranet, confluence pages, data sources from call centers,
- How good is the data quality itself (e.g. we see often low text content in websites, or inconsistent maintenance such as missing titles and structure which are key for a good chatbot or search)
- Is the data/content owner identified in your company? Or are several owners of topics scattered around? Identify them and list them together. Involve these stakeholders in your Gen. AI project from the beginning!
5. Following AI Governance Principles: Are you adhering to AI ethics principles with your solution?
6. Unique Value Proposition: Why is your solution better than others in the market? (e.g. you can faster extract data, or you can search within more information context or your solution is more straightforward to use, hence has less entry hurdles?). Add a slogan to your solution: “ChatGPT to summarize legal docs.”
5. Data Source: Outline what data you have or need to obtain to make the solution work. Define how much and quality of data you have and who ‘owns’ it.
6. Value Streams: Describe how your concept drives value – estimate your saving potential (ROI in monthly hours of work) and revenue potential due to a new market or additional service you can provide thanks to Gen. AI.
7. Cost Structure: Lay out the major costs and expenses necessary to build and run your solution. Here we will provide an additional in-depth article on the costs related to Generative AI solutions.
8. AI Ethics and Governance: Write down any ethical, legal, or compliance hurdles you’ll need to manage.
9. Key Metrics: Outline the essential metrics you will use to track the success and growth of your solution - leading/lagging metrics that reflect the overall health and success of your venture. There are metrics that play a role especially with llm solution such as:
- How reliable is the system (hallucinations, accuracy score, llm evaluation metrics)? This helps you to build trust with your customers which is essential with llms.
- How much efficiency can you create with your product? Measured in cost analysis
- How transparent is your product? Again outline how your product is build, which data base you use
Which Generative AI use cases are bringing high value?
Most companies currently focus on optimizing efficiency when it comes to finding relevant information. This major use case includes extracting relevant data from multiple sources like confluence, notion, emails, pdfs or websites. Why is this case approached by major enterprises and SME's around the world?
- Data content can be extensively tested, optimized and amended so that your generative AI application works perfectly together with your underlying data sources
- Companies can make first experiences regarding Generative AI costs without exposing to a larger group of clients
- Image risks due to hallucinations (e.g. generated text) can be reduced by using standard llm evaluation metrics.
Thus, before providing your Gen. AI product client-facing you it can be substantially improved.
Need support with your Generative AI Strategy and Implementation?
🚀 AI Strategy, business and tech support
🚀 ChatGPT, Generative AI & Conversational AI (Chatbot)
🚀 Support with AI product development
🚀 AI Tools and Automation
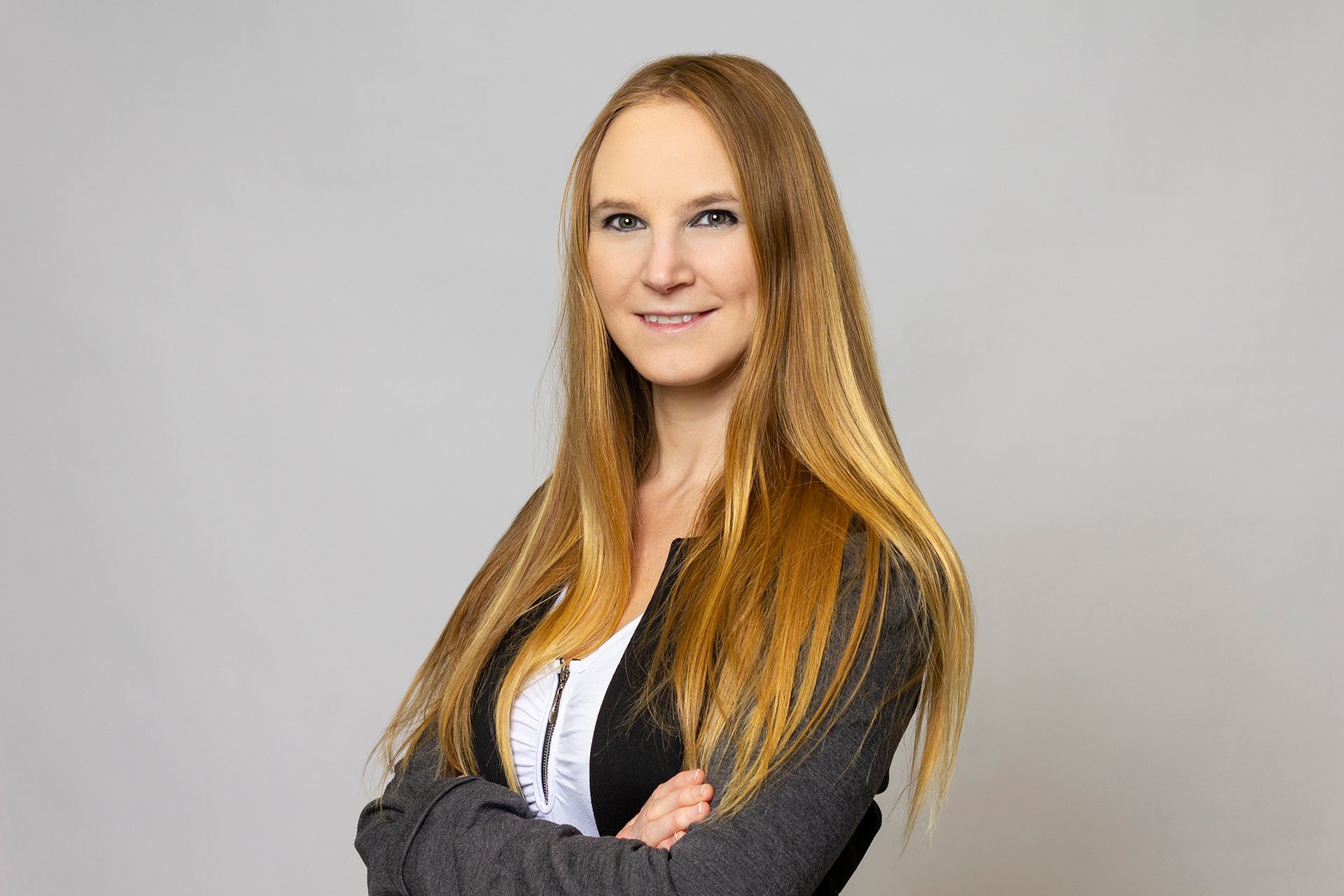
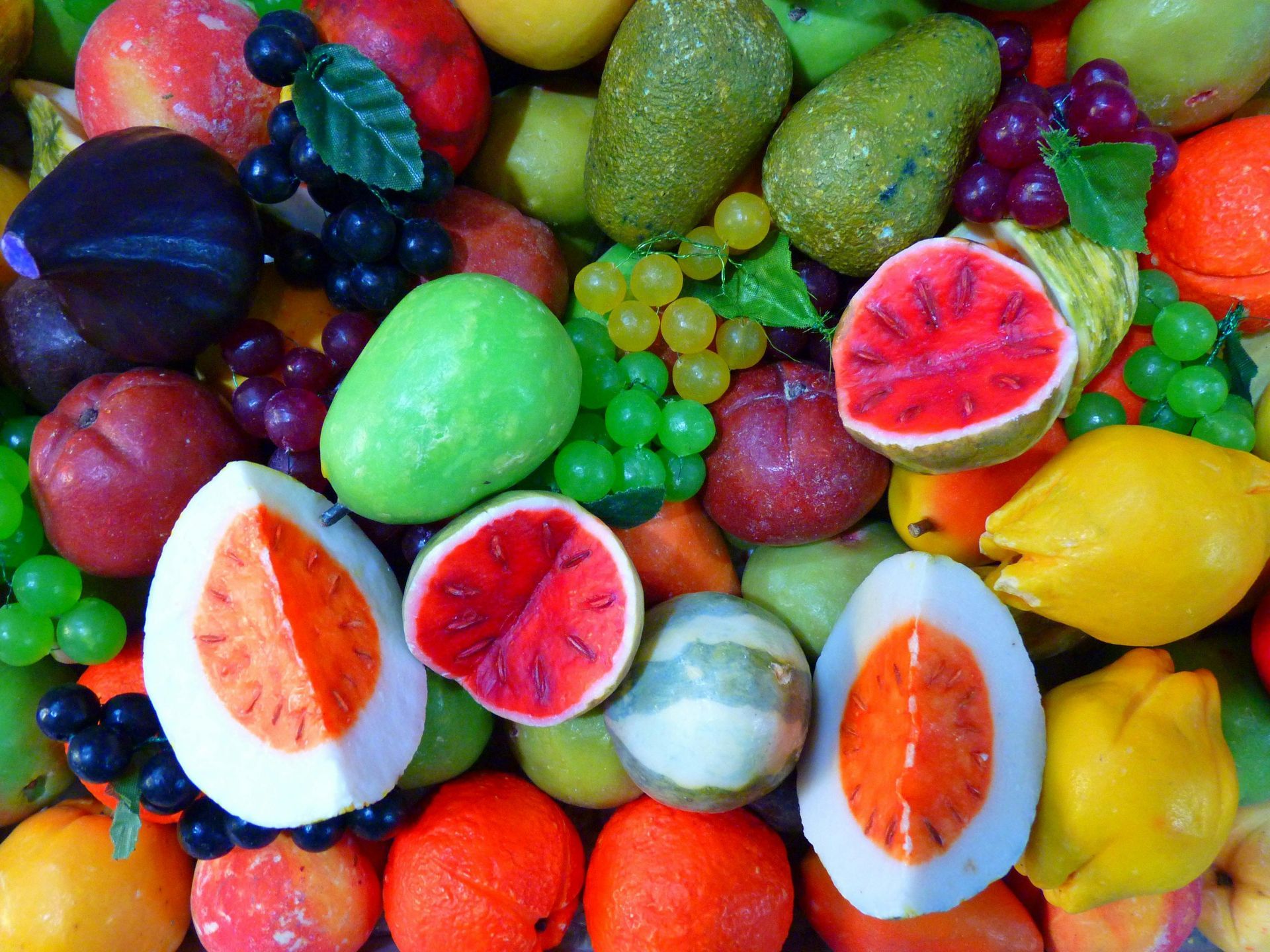
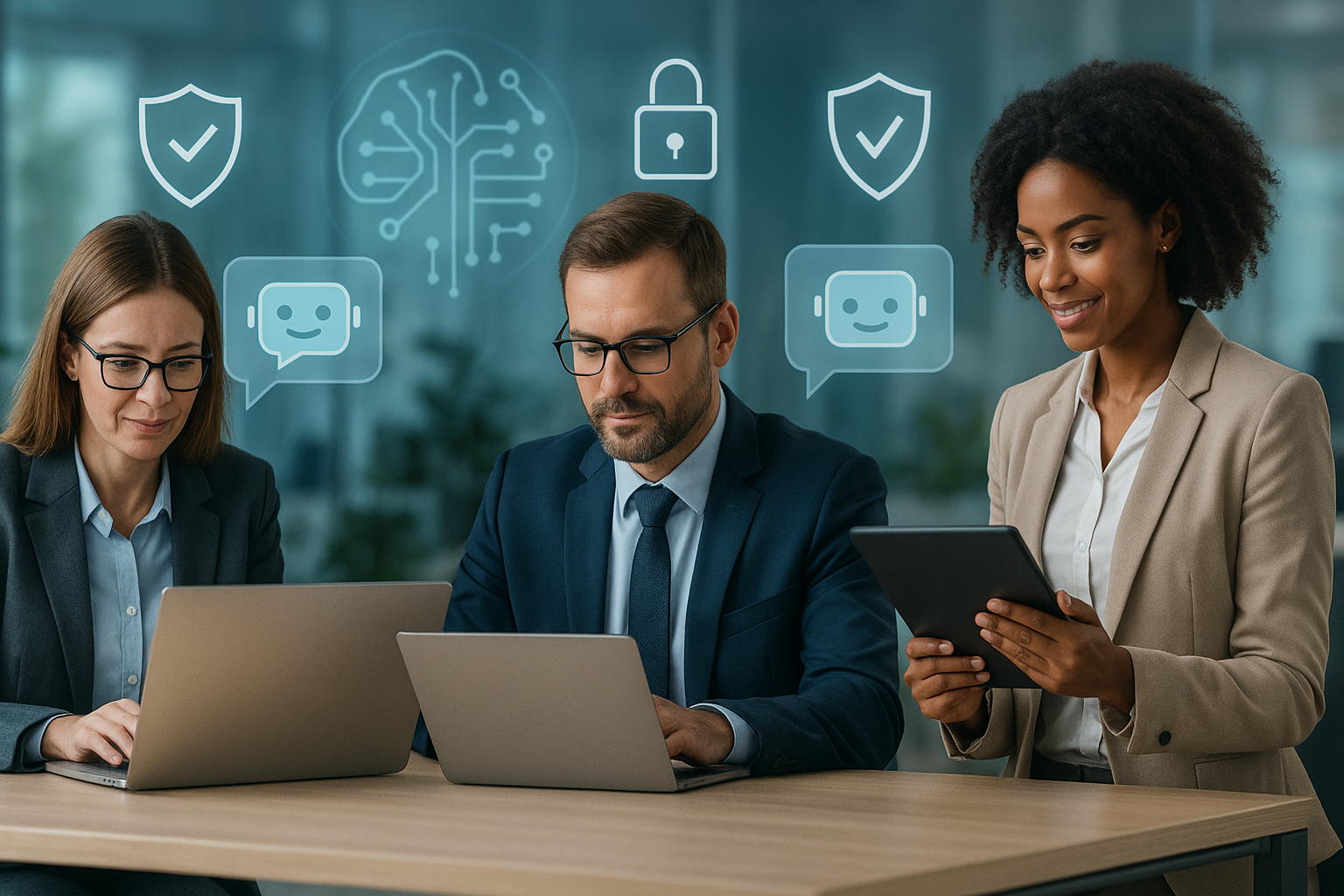
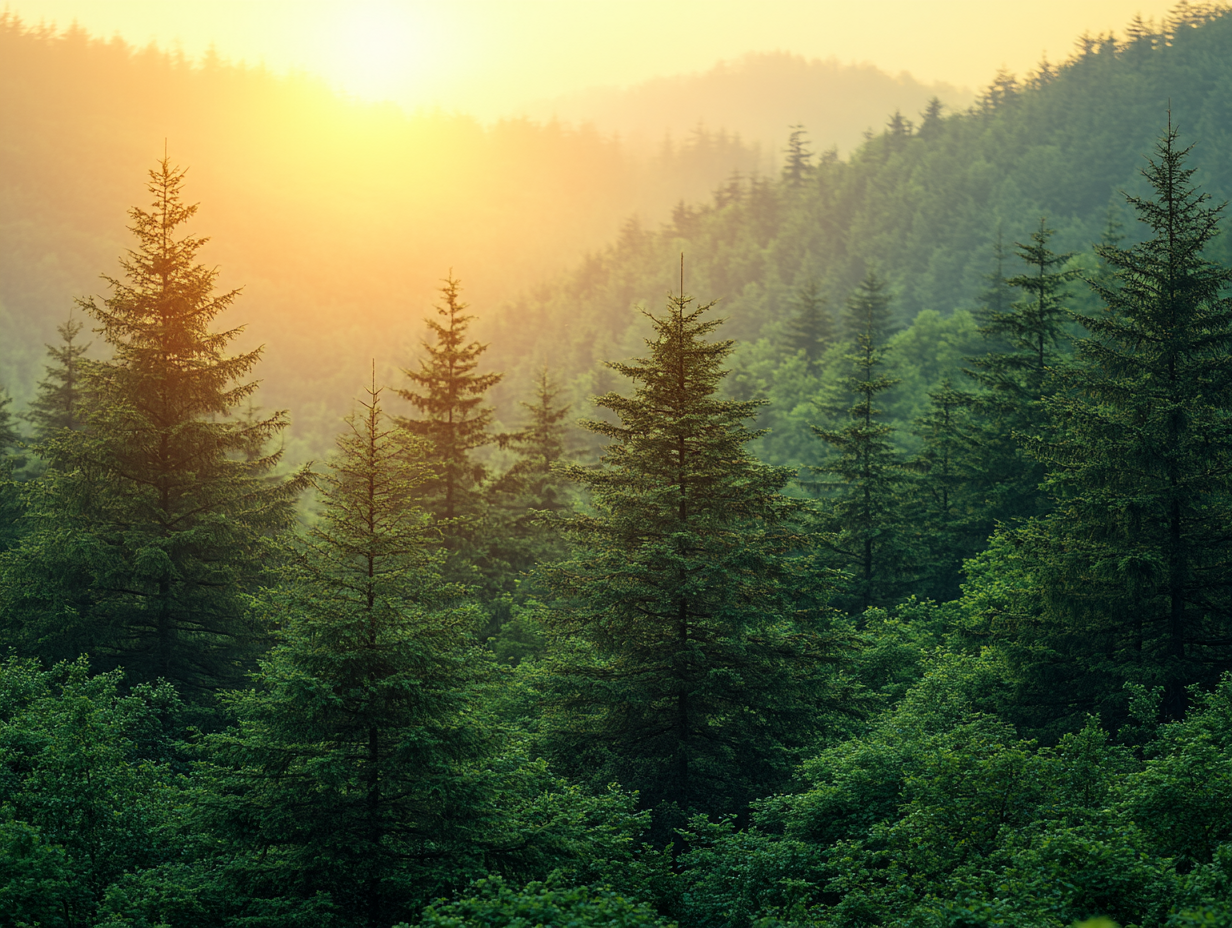